In New York on October 9, 2023, researchers from the Icahn School of Medicine and the University of Michigan discovered machine learning models used in healthcare. They found that when these models are very successful, they can face problems.
Their study looked at how using predictive models can change how healthcare is provided and affect the original training of the models. Surprisingly, in some cases, this adjustment can make the models perform worse.
First and corresponding author Akhil Vaid, MD, Clinical Instructor of Data-Driven and Digital Medicine (D3M), part of the Department of Medicine at Icahn Mount Sinai, said, “We wanted to explore what happens when a machine learning model is deployed in a hospital and allowed to influence physician decisions for the overall benefit of patients.”
“For example, we sought to understand the broader consequences when a patient is spared from adverse outcomes like kidney damage or mortality. AI models possess the capacity to learn and establish correlations between incoming patient data and corresponding outcomes, but the use of these models, by definition, can alter these relationships. Problems arise when these altered relationships are captured back into medical records.” he added.
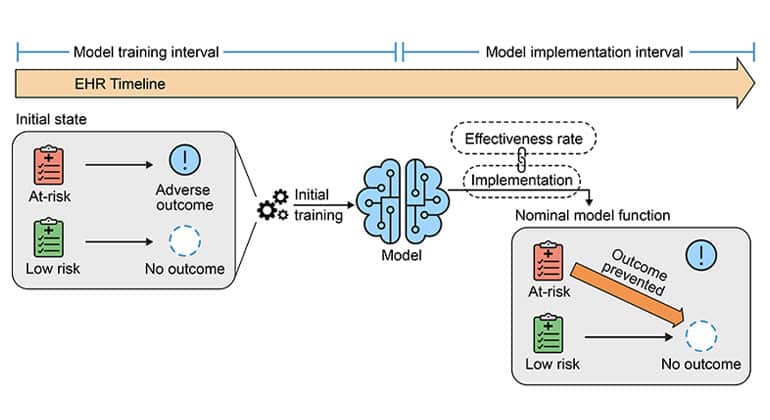
The study looked at two big hospitals, Mount Sinai Health System in New York and Beth Israel Deaconess Medical Center in Boston, and they studied 130,000 cases where people needed critical care. The researchers focused on three essential situations:
- Retraining the Model:Â Usually, we retrain these computer models to make them better over time. It’s like giving them new lessons. However, the study found that sometimes, this makes the models worse. It’s like messing up what the models already learned.
- Creating a New Model:Â When the model predicts something terrible might happen, it can help save lives. For example, it can predict sepsis, which is a severe infection. But if the model starts preventing sepsis, it might also prevent the death that comes after it. So, if we make a new model later on to predict death, it might also get confused because we changed the relationships between things. In simple words, it’s like changing the rules of a game, and then it’s hard for everyone to play by the new regulations.
- Simultaneous Use of Two Predictive Models: When two models make predictions simultaneously, relying on one set of forecasts makes the other useless. This means that to make accurate predictions, we need to use the latest data, which can be expensive or complicated.
The co-senior author, Dr. Karandeep Singh, said, “Their findings show how hard it is to keep these predictive models working well in real medical situations. If the group of patients changes, the model’s performance can drop significantly. And even if you try to fix it, those fixes only work if you pay attention to what the models learn from the data. It’s like trying to use two maps simultaneously, but one becomes useless, and you must ensure the maps are current.”
“We shouldn’t think that predictive models are unreliable,” said Dr. Girish Nadkarni, an expert in medicine and data at Mount Sinai. Instead, we should remember that these tools need regular attention and understanding. They will only be effective if we pay attention to how well they’re working and use them carefully. Think of them like any other medical tool – they require care. Using them without thinking might give us wrong information, make us do unnecessary tests, and cost us more money.
Dr. Vaid adds “that health systems should monitor how these models affect people and that government agencies should make guidelines. These lessons aren’t just for healthcare – they apply to any predictive model. In our world, models can affect each other, so we need to be smart about how we use them. If we’re not careful, we might make models useless.”
The research paper is “Implications of the Use of Artificial Intelligence Predictive Models in Health Care Settings: A Simulation Study.”
In conclusion, this study shows that using predictive AI in healthcare is powerful, but it’s like handling a delicate tool. If we don’t monitor how well these AI models are doing and update them carefully, they might not work as we want. When the kinds of patients change, the AI models might struggle to make accurate predictions. So, it’s crucial to use these models wisely and monitor them regularly to avoid mistakes and unnecessary costs in healthcare.
Journal reference:
- Akhil Vaid, Ashwin Sawant, et al., Implications of the Use of Artificial Intelligence Predictive Models in Health Care Settings. Annals of Internal Medicine. DOI: 10.7326/M23-0949.