Indeed, the explosive characteristics of perchlorate have been a focal point in chemical experiment safety education, underscoring the potential dangers associated with shocks or heat. It’s important to note that complex ions, especially those containing organic groups or other oxidizable atoms, can also display explosive properties. This highlights the importance of carefully understanding and handling these compounds to prevent unexpected chemical experiment accidents.
A new study aimed to identify the specific molecular/crystal structure responsible for their explosive properties.
The Hirschfeld surface analysis method has been widely used for visualizing and quantifying the crystal structure and molecular interactions of compounds. Despite its advantages, these methods rely on human judgment, limiting their effectiveness. Recent studies have explored integrating deep learning and artificial intelligence (AI) to overcome these limitations.
Led by Professor Takashiro Akitsu from the Tokyo University of Science, a team of researchers utilized deep learning to analyze the Hirschfeld surface of salen-type metal complexes. This approach aims to leverage AI to uncover features that might be challenging for human observers, enhancing the potential of Hirschfeld surface analysis.Â
Prof. Akitsu said, “Salen-type complexes are an emerging and lucrative area of research, primarily due to their diverse functions. Actual experiments on explosive and thermal properties of these materials are accurate but extremely dangerous, and therefore, using AI to study these properties by solely analyzing the crystal structure can be quite advantageous.”
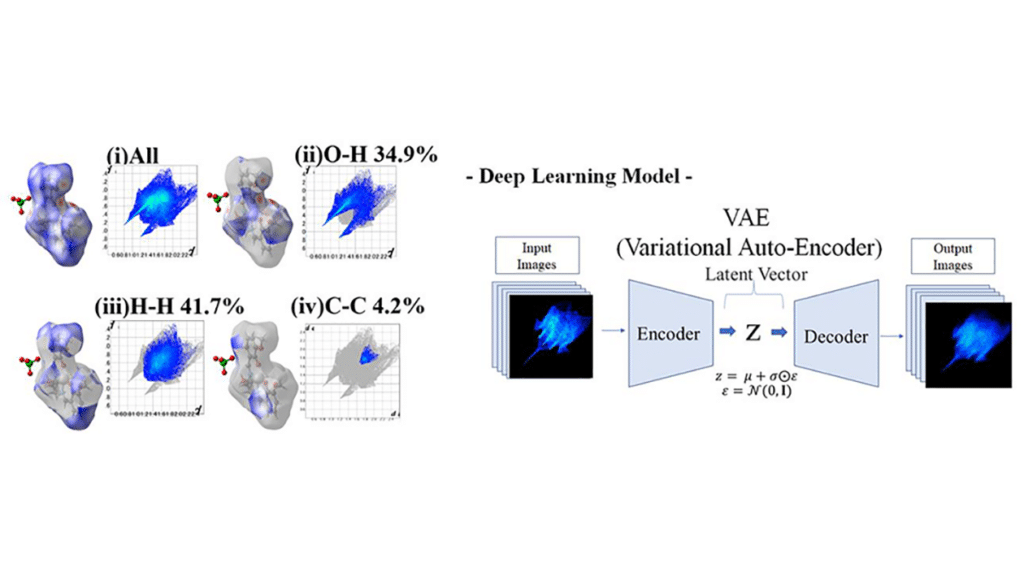
The research team generated extensive datasets of Hirschfeld fingerprint plots for sale-type metal complexes from the Cambridge Crystal Database (CCDC). They employed deep learning techniques to investigate the crystal structure features contributing to the explosiveness of these complexes.Â
Specifically, a special variational autoencoder was used to transform information from the fingerprint plot images into a low-dimensional vector. This allowed for quantitative analysis of plot shapes, previously undertaken qualitatively.
The findings indicated that salen-type metal complexes lack distinctive structural features, suggesting that their explosive nature is associated with the chemical bonding of perchlorate ions and their surrounding intermolecular interactions.
Highlighting the importance of this study, Prof. Akitsu explains, “Traditionally, the field of crystal engineering has concentrated solely on the interactions of small molecules in crystals. But in the future, interactions in complex systems will gain significance.”
“This means that studying their intermolecular interactions will become even more crucial. Our novel method can help study such interactions by understanding the crystal structure alone. Moreover, it can also contribute to discovering new drugs and advancing catalytic research.”
This study highlights the underutilization of the Cambridge Crystal Database (CCDC) despite its vast collection of over 1 million entries. The innovative method proposed in the research provides insights into the explosive nature of perchlorates and encourages the broader use of this database.
By promoting a safer, data-driven approach to studying the physical properties of compounds, the study contributes to the advancement of crystal engineering and research in energetic materials. Overall, this research opens avenues for discovering new and interesting combinations, leveraging the wealth of information within the CCDC.
Journal Reference:
- Takashiro Akitsu, Yuji Takiguchi, Shintaro Suda, Daisuke Nakane et al. Deep-learning prediction of safety moiety of salen-type complex crystals towards explosive perchlorate salts. FirePhysChem. DOI: 10.1016/j.fpc.2023.12.004