Obstructive sleep apnea is a potentially serious sleep disorder. It causes breathing to stop and start during sleep repeatedly. It is estimated that up to one billion people worldwide suffer from obstructive sleep apnoea, and the number is expected to grow due to population aging and increased prevalence of obesity.
The identification of sleep stages is necessary for the diagnostics of sleep disorders, among which obstructive sleep apnea (OSA) is one of the most prevalent.
Traditionally, it was believed that during sleep, the body moves through five different stages: rapid eye movement (REM) sleep and three stages of non-REM sleep. However, manual scoring of sleep stages is time-consuming, subjective, and costly.
To overcome this shortcoming, scientists from the University of Eastern Finland have devised an accurate deep learning approach for automatic classification of sleep stages. Their model could also study the effect of OSA severity on the classification accuracy.
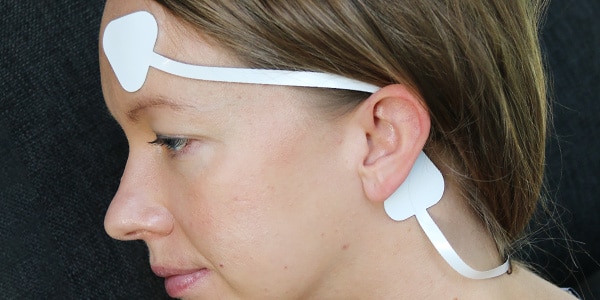
To develop this model, scientists used polysomnographic recording data from healthy individuals and individuals with suspected OSA. During tests, scientists found that the model can identify sleep stages with an 83.7% accuracy when using a single frontal electroencephalography channel (EEG), and with an 83.9% accuracy when supplemented with electrooculogram (EOG).
On the other hand, the model achieved accuracies of 82.9% (single EEG channel) and 83.8% (EEG and EOG channels) in patients with suspected OSA. The single-channel accuracies ranged from 84.5% for individuals without OSA to 76.5% for severe OSA patients.
Scientists noted, “The deep learning enables automatic sleep staging for suspected OSA patients with high accuracy.”
The study was published in the IEEE Journal of Biomedical and Health Informatics.