From the beginning of the COVID-19 pandemic, many research groups worldwide turned their attention to SARS-CoV-2 and, in particular, to the immune response to infection and vaccination. Since 2020, thousands of human monoclonal antibodies to SARS-CoV-2 have been isolated and characterized.
A new study shows that it is possible to use the genetic sequences of a person’s antibodies to predict what pathogens those antibodies will target. As reported in the journal Immunity, the new approach successfully differentiates between antibodies against influenza and those attacking SARS-CoV-2, the virus that causes COVID-19.
“Our research is in a very early stage, but this proof-of-concept study shows that we can use machine learning to connect the sequence of an antibody to its function,” said Nicholas Wu, a professor of biochemistry at the University of Illinois Urbana-Champaign who led the research with U. of I. biochemistry Ph.D. student Yiquan Wang; and Meng Yuan, a staff scientist at Scripps Research in La Jolla, California.
“Although information of many human monoclonal antibodies to SARS-CoV-2 is now publicly available, it has been difficult to leverage all available information to investigate public antibody responses to SARS-CoV-2. One major challenge is that the data from different studies are rarely in the same format. This inconsistency imposes a huge barrier to data mining.”
“The establishment of the coronavirus antibody database (CoV-AbDab) has enabled researchers to deposit their antibody data in a standardized format and has partially resolved the data formatting issue. However, not every SARS-CoV-2 antibody study has deposited its data to CoV-AbDab. Furthermore, IGHD gene identities, nucleotide sequences, and donor IDs are not available in CoV-AbDab, making it challenging to study public antibody responses using CoV-AbDab. Thus, additional efforts must be made to fully synergize the information across many different SARS-CoV-2 antibody studies to investigate and decipher public antibody responses.” Study quotes.
With enough data, scientists should be able to predict not only the virus an antibody will attack but which features on the pathogen the antibody binds to, Wu said. For example, an antibody may attach to different parts of the spike protein on the SARS-CoV-2 virus. Knowing this will allow scientists to predict the strength of a person’s immune defense, as some targets of a pathogen are more vulnerable than others.
Wu said that the new approach was made possible by the abundance of data related to antibodies against SARS-CoV-2.
“In 20 years, scientists have discovered about 5,000 antibodies against the flu virus,” he said. “But in just two years, people have identified 8,000 antibodies for COVID. This provides an opportunity that’s never been seen before to study how antibodies work and to do this kind of prediction.”
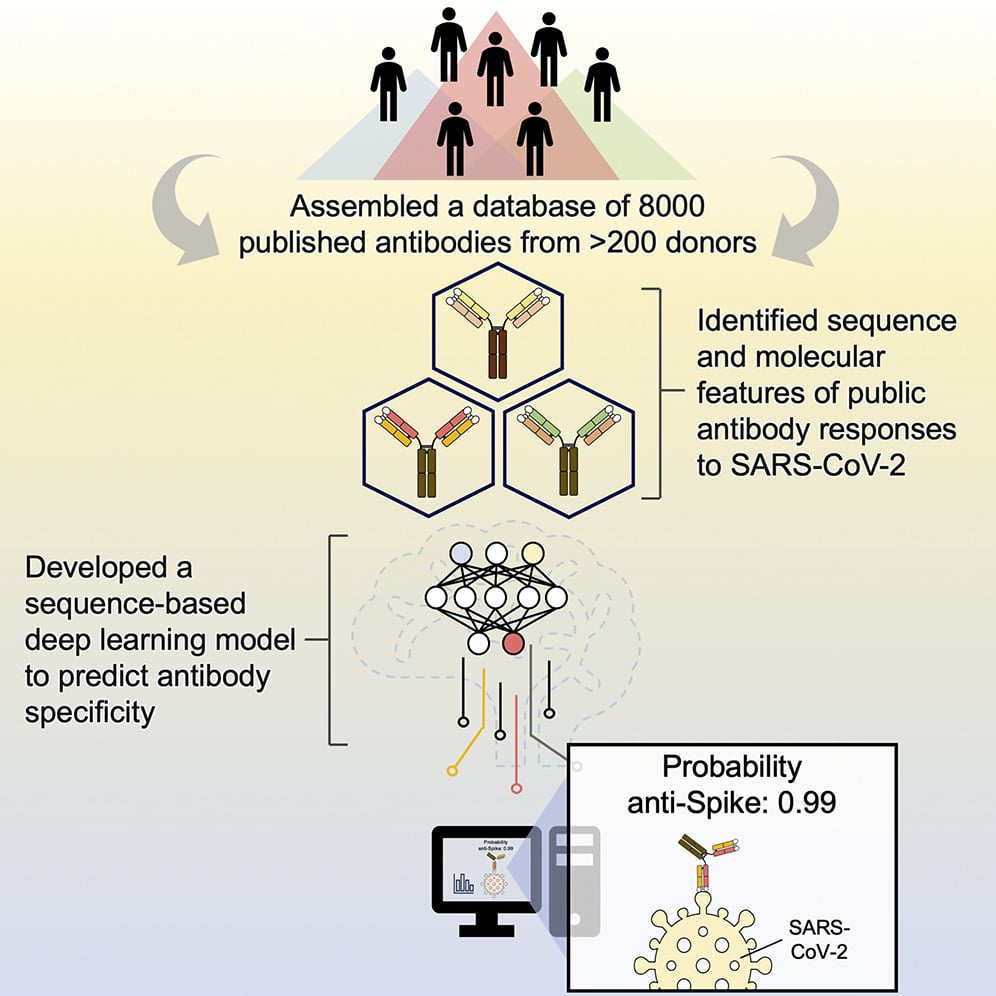
Highlights
- Assembled a dataset of ∼8,000 published antibodies to SARS-CoV-2 S from >200 donors
- Antibodies to RBD, NTD, and S2 have distinct convergent sequence and molecular features
- Public antibody clonotypes show recurring affinity maturation pathway
- Provided a proof of concept for antibody specificity prediction using deep learning
The model was designed to distinguish whether the sequences coded for antibodies targeting regions of the influenza virus or on the SARS-CoV-2 virus. The researchers then checked the accuracy of those predictions.
“The accuracy was close to 85% overall,” Wang said.
“I was actually quite surprised that it worked so well,” Wu said.
The team is working to improve its model to more precisely determine which parts of the virus the antibodies attack.
“If we can make these predictions based on antibody sequence, we might also be able to go back and design antibodies that bind to specific pathogens,” Wu said. “This is not something that we can do now, but those are some implications for future study.”
Limitations of the study:
Many antibodies in our collection were isolated from SARS-CoV-2-infected individuals. However, sequence information of the infecting viral variants was not available in the original publications.
Although most of these antibodies were isolated during the early phase of the COVID-19 pandemic, some antibodies in our collection may have been elicited by a SARS-CoV-2 variant rather than the ancestral Hu-1 strain. Relatedly, this study did not examine the antibody specificity to different variants. Future analysis could investigate the relationship between antibody sequence features and neutralization breadth by leveraging the published information on antibody neutralization activity to different variants.
Journal Reference
- Yiquan Wang, Meng Yuan, Huibin Lv, Jian Peng, Ian A. Wilson, Nicholas C. Wu; A large-scale systematic survey reveals recurring molecular features of public antibody responses to SARS-CoV-2, Immunity DOI: 10.1016/j.immuni.2022.03.019